The Decline Of Statisticians In Big Data: A Deep Dive
Is the venerable statistician, once the gatekeeper of data insights, facing an unprecedented challenge in the age of Big Data? The data clearly suggests a shift in the spotlight, with the rise of data scientists seemingly eclipsing the traditional role of statisticians. This transition raises crucial questions about the evolving landscape of data analysis and the skills needed to thrive in it.
The digital realm is awash with data. Every click, every transaction, every interaction leaves a digital footprint, creating an unprecedented deluge of information. Google Trends, a barometer of public interest, paints a compelling picture. While the popularity of "Big Data" continues its meteoric rise, the search interest in "statistician" appears to be waning, suggesting a potential marginalization of the profession. This trend is a focal point of discussion within the data science community, a space where professionals actively engage in debates and discussions, seeking to understand and adapt to the changing environment. The core question then becomes: what forces are at play, reshaping the landscape of data analysis and reshaping the roles of those who work in it?
The article The Risky Eclipse of Statisticians provides a thought-provoking analysis of this dynamic. It highlights the perceived shift in the data analysis field, which is increasingly influenced by data scientists. The core argument presented in this article seems to suggest a notable shift. But to grasp the significance of this transformation, its crucial to understand the distinct characteristics of these two professions.
Aspect | Statistician | Data Scientist |
---|---|---|
Primary Focus | Designing and conducting studies, analyzing data to identify trends, and drawing inferences based on statistical principles. | Extracting insights from large and complex datasets, building predictive models, and communicating findings to a wide audience. |
Core Skills | Statistical theory, experimental design, probability, regression analysis, hypothesis testing. | Machine learning, programming (Python, R), data visualization, database management, communication and storytelling. |
Tools | Statistical software (SAS, SPSS, R), mathematical modeling, and data analysis techniques. | Python, R, SQL, Hadoop, Spark, Tableau, and various machine learning libraries. |
Typical Tasks | Analyzing surveys, designing experiments, calculating probabilities, drawing conclusions from samples, and developing reports. | Data mining, model building, data visualization, predictive analytics, and presenting findings to stakeholders. |
Educational Background | Advanced degree in statistics, mathematics, or a related field. | Advanced degree in statistics, computer science, mathematics, or a related field, or professional certifications. |
Philosophical Approach | Emphasis on rigor, accuracy, and statistically sound methodologies. | Focus on practical application, and identifying trends and actionable outcomes. |
Career Trajectory | Researcher, data analyst, consultant, professor, and actuary | Data Analyst, Machine learning engineer, data architect, and business analyst. |
For further insights and detailed comparative analysis, consult the official website of the American Statistical Association https://www.amstat.org/
Historically, statisticians have been the leaders of data, using sophisticated mathematical methods to extract meaningful insights. They have shaped everything from medical research to market analysis, ensuring that decisions are based on rigorous data. Yet, the rise of Big Data has brought with it an explosion of data volumes, velocities, and varieties. This has, in turn, created the need for new skill sets. Data scientists emerged, armed with the tools of programming, machine learning, and data visualization, capable of navigating these enormous datasets to extract insights that are rapidly applicable. This is not to say that statistics has become irrelevant. The core principles of statistical thinking, such as inference, remain critical. But the emphasis has shifted: today's data analysts need more than just a deep understanding of mathematics; they also need to be proficient in computer programming, data visualization, and effective communication. They need to be able to quickly process information, generate predictive models, and tell stories.
The data science community, boasting over 1.3 million subscribers in its online discussions and platforms, is a testament to this new ecosystem. It reflects the evolution of data-driven decision-making. The conversations that take place within this group and many others, the articles shared by Ritika Trikhla and the discussions on platforms such as Hacker News (with over 78,000 subscribers), highlight the challenges and opportunities in this dynamic environment. There is a recognized need to not only understand the data but also to be able to use it in making predictions, decisions, and presentations.
A core consideration in this transition is the availability of education and training, and how it is adapted to the evolution of data science. Many educational institutions and training programs have adapted by offering more courses in machine learning and other computer science topics. This has resulted in an education model that is more multidisciplinary, and one that reflects the skills that modern data analysts need. This training is crucial, not just for those wanting to enter the field, but also for people looking to enhance their capabilities in the current environment.
The rise of data science also poses important questions about the broader principles of ethics and responsible use of data. As we become increasingly reliant on the use of data to make decisions, concerns regarding bias, privacy, and fairness become even more critical. Data scientists and statisticians must be educated to consider ethical principles and their responsibilities to these areas.
The evolving data landscape is also changing how industries operate. From healthcare and finance to marketing and education, companies are integrating data science to drive efficiency, inform strategies, and improve outcomes. One such example is "Eclipse Risk Management (eRisk)", which utilizes data-driven approaches to enhance risk assessment. The focus on real-time insights, dynamic data, and rapid responses, highlights the increased demands for expertise in data analysis.
The emergence of new tools and technologies has accelerated this transformation. The rise of cloud computing platforms, open-source software, and advanced machine-learning algorithms have increased the ability of data scientists to solve complex problems. The evolution of data analysis is not a story of displacement, but one of integration. Statisticians and data scientists are converging and complementing each other's expertise. With a solid foundation in statistical principles, a strong understanding of mathematics and computer science, and the capacity to combine the two, the skills of a modern data professional are always in demand.
In conclusion, the risky eclipse does not necessarily signify the decline of statistics, but rather the emergence of new, interdisciplinary fields within data analysis. The skills that have been traditionally associated with statisticians are still critically important, and must be integrated with programming, data visualization, machine learning, and strong communication skills, to succeed in the modern data-driven era. The field is moving forward and will continue to adapt. The focus is on innovation, a constant pursuit of insight and understanding. It is critical that we foster an environment for those who are interested in, and fascinated by, data. Data professionals will remain indispensable partners in the journey to insight, driving us toward a future informed by data.
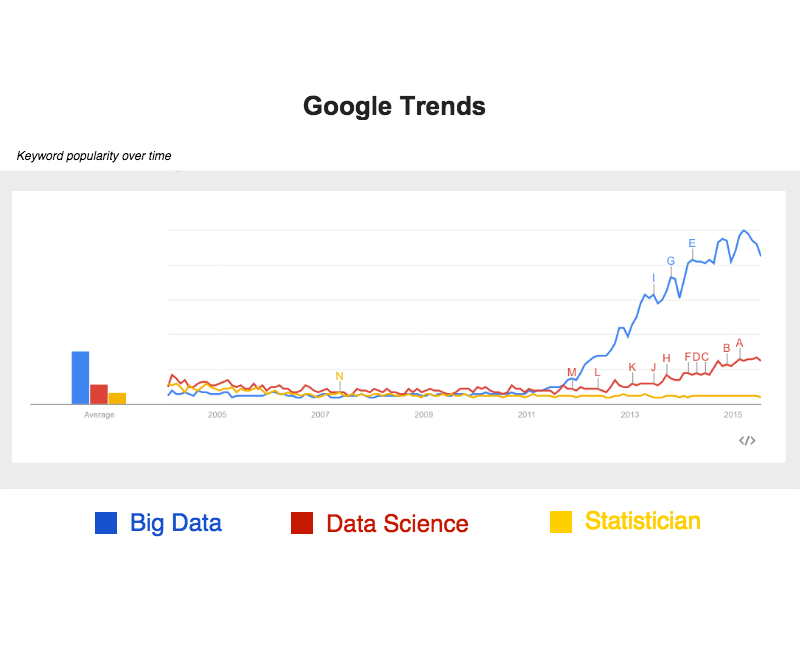
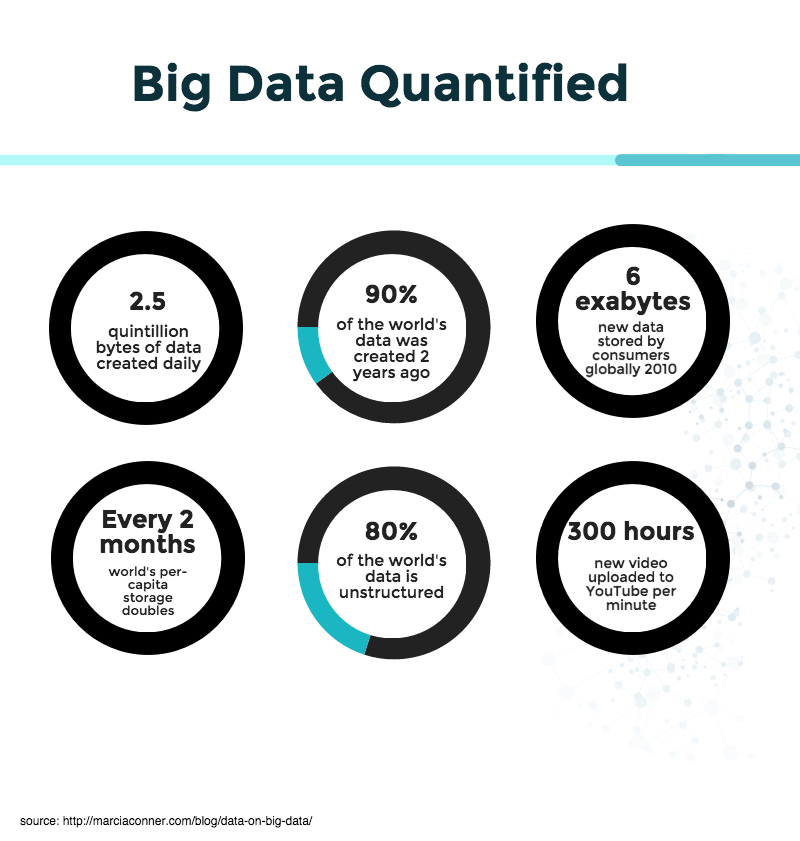
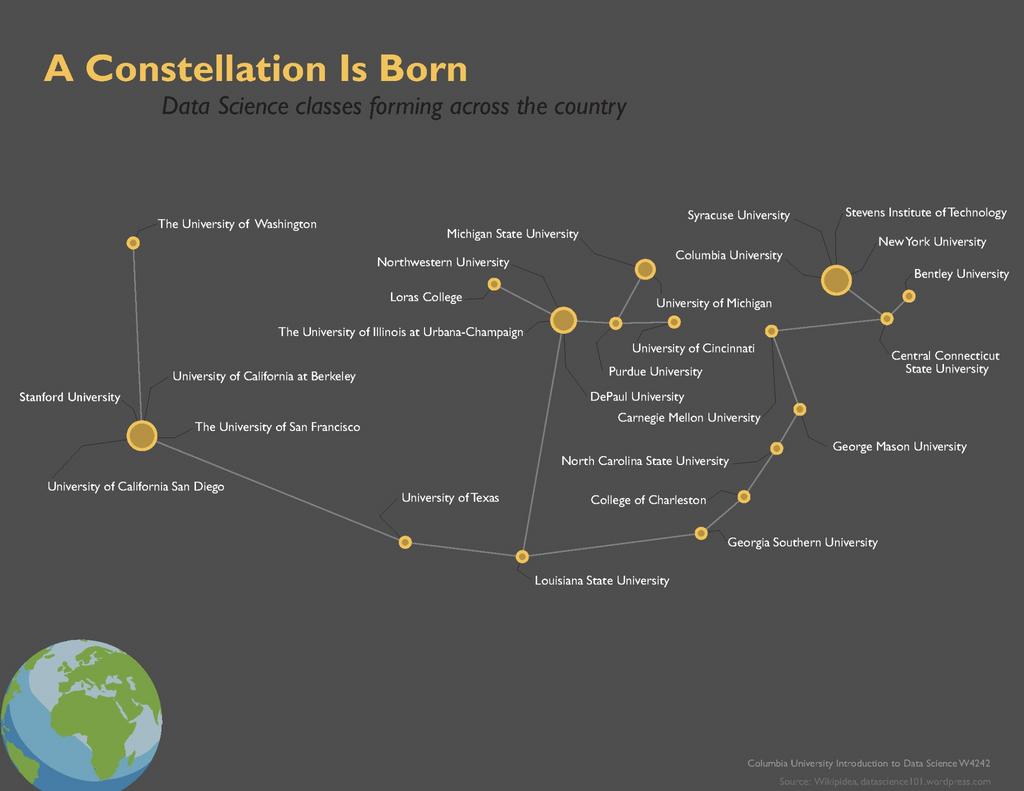